Machine learning is a rapidly evolving technology that has seen a surge of interest in recent years, and the logistics and supply chain industry is no exception. As logistics become more complex and global, businesses are turning to the emergent technology of machine learning to unlock new levels of efficiency and accuracy. Take the time out now to learn how machine learning in logistics can help your business reach new heights. Read on to find out more.
From manual processes to automated freight management, DFreight is revolutionizing how goods are shipped worldwide. As a digital freight forwarder with an online platform and mobile app and using new technologies such as machine learning in logistics, DFreight is leading the way in the future of freight forwarding.
Table of Contents
What is Machine Learning?
Machine learning is a type of artificial intelligence that uses algorithms to enable computers to learn and take decisions from data. Machine learning is a type of technology that allows a system to learn from large quantities of data and then make decisions based on what it learns.
Exploring the Use of Machine Learning in Logistics
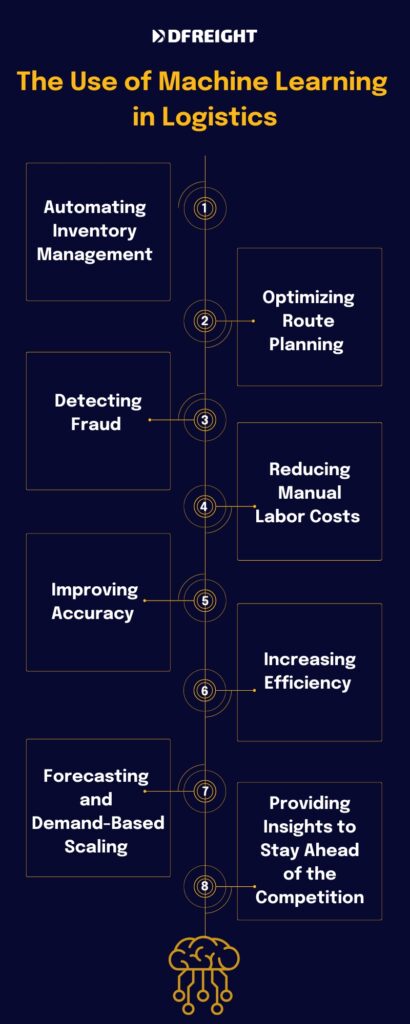
Logistics is an ever-changing dynamic – and one of the most important factors of successful operations for businesses. Many companies have leveraged new technologies such as machine learning (ML) in order to help streamline operations and increase their bottom line.
Exploring the use of machine learning in logistics can be beneficial in several different ways. For example, ML can be used to automate inventory management, optimize logistics planning, detect fraud, and more. ML can reduce manual labor costs by automating decision-making processes that would traditionally require human analysis. It can also improve accuracy by streamlining operations resulting in fewer mistakes. ML also allows for quicker and more knowledgeable decisions which can improve the efficiency of overall logistics operations.
Furthermore, leveraging machine learning in logistics can help with forecasting and demand-based scaling. By using machine learning to analyze customer buying trends, companies can better project future demand as well as prepare for possible disruptions in inventory and supply chain. With better forecasting capabilities, companies can better adjust their services to meet customer requirements faster.
Overall, exploring the use of machine learning in logistics has the potential to benefit companies in numerous ways. By automating labor-intensive processes, improving accuracy, and allowing companies to better forecast the future, they can better manage their businesses with increased efficiency. ML also provides companies with the insights needed to stay ahead of the competition. Companies that take advantage of ML can be poised to succeed in the competitive logistics market.
Benefits of Machine Learning for Logistics Operations
Machine learning technology can be used to improve many aspects of a logistics operation. It can automate and streamline manual tasks such as order management, order fulfillment, shipment tracking, and route optimization. Additionally, it can be used for predictive analytics, allowing companies to anticipate customer needs and respond quickly and effectively. Finally, machine learning can help reduce errors, since it is more reliable than manual data entry and offers more accurate predictions.
Challenges of Implementing Machine Learning in Logistics
Though machine learning can provide significant benefits, it can also be challenging to implement. The technology requires a robust data architecture and a large amount of data to be able to identify patterns and make accurate predictions. Additionally, machine learning algorithms are complicated and require skilled personnel to set up and maintain. Finally, the cost of implementing machine learning systems can be prohibitive for some companies, especially smaller ones.
Real-World Examples of Machine Learning in Logistics
There are many real-world examples of how machine learning is being used to improve efficiency within the logistics industry.
Amazon: Amazon uses machine learning in its logistics operations to help optimize delivery routes, reduce costs, and improve customer satisfaction. Amazon uses an AI-powered system that considers current and forecasted traffic, product specifications, delivery preferences, and customer preferences to optimize delivery routes. This system is called Amazon Logistics Vision and is currently deployed in the US, Europe, Japan, and India.
Nike: Nike is using machine learning to optimize its transportation networks for faster and more efficient delivery of its products. The company’s AI and ML-powered Nike Speed Platform is an intelligence-driven system that consolidates data from supply chain partners across the globe to power faster shipping. The system uses predictive analytics to understand customer demand and quickly provide a response.
Tesla: Tesla applies AI and machine learning to almost every aspect of its business, including logistics. The company uses complex algorithms to plan routes and maximize the efficiency of its delivery network. The algorithms prioritize routes based on traffic patterns, weather conditions, and customer orders.
McDonald’s: McDonald’s implemented its machine learning-driven AI Planner to optimize its supply chain and logistics operations. The system uses statistical modeling and predictive algorithms to minimize warehouse inventory, streamline routing, and reduce costs. McDonald’s has also incorporated AI-driven drones to automate end-to-end delivery operations.
Apple: Apple‘s machine learning platform, Core ML, allows for advanced data analysis and integration of machine learning-powered tools into operations. Core ML is used in logistics and supply chain management to optimize inventory forecasts and provide predictive analytics for demand prediction. Apple’s fleet of robots and drones provides an efficient delivery experience, while the ML models allow for adaptive routing and faster delivery.
IKEA: IKEA uses machine learning algorithms to optimize its supply chain operations. The company uses predictive models to anticipate customer demand and thus allocate and prioritize inventory to stores accordingly. This helps the company better manage costs, reduce stock-outs and give resources to the right places at the right time. Additionally, through machine learning, IKEA can efficiently issue orders and track shipments, thus ensuring an efficient supply chain process.
Intel: Intel integrates machine learning algorithms into its supply chain operations to automate and optimize the transport of products from warehouses to retailers. With machine learning, Intel is able to anticipate demand and use predictive models to anticipate when to move products to various regions in the world. In addition, this helps Intel to optimize the cost of shipping, transportation, and delivery of the goods.
Adidas: Adidas uses machine learning to optimize its transport of goods from its manufacturer to the warehouse. With machine learning, Adidas can predict potential outflows and stock-outs while helping to optimize costs and transport times. Adidas also uses machine learning algorithms to assess trends and predict the impact different shipping methods have on the overall delivery cost.
Samsung: Samsung utilizes machine learning algorithms to track and process the complex logistics associated with its products. By using machine learning to deliver real-time insights into shipping costs and times, Samsung can streamline its processes and ensure the timely delivery of products to customers. Additionally, through machine learning, Samsung can allocate resources better to increase the efficiency of its supply chain.
Coca-Cola: Coca-Cola has successfully implemented machine learning into its logistics operations to understand consumer behavior better and predict future demands. This helps Coca-Cola effectively manage its transportation and delivery operations while ensuring that suitable beverages are available in the right locations to meet customer expectations. In addition, machine learning also helps Coca-Cola with forecasting, better cost management, and increased overall efficiency.
Schneider Electric: Schneider Electric has successfully integrated machine learning algorithms into its logistics operating system. The company utilizes various predictive models to automate and streamline its delivery operations while also helping optimize the cost of transporting goods. Furthermore, Schneider Electric has implemented machine learning algorithms to anticipate consumer demand, allowing them to manage their supply chain and resources better.
Pepsi: Pepsi has successfully integrated machine learning into its logistics operations to leverage predictive analytics. By using machine learning algorithms, Pepsi can anticipate demand and optimize the delivery of its products. With this, Pepsi can identify the most efficient shipment routes while reducing delivery costs.
Future Applications of Machine Learning in Logistics
Machine learning technology is likely to be used for more complex tasks in the future, such as predicting customer demand and forecasting inventory levels. Additionally, it could be used to optimize delivery routes, reduce fuel consumption, and automate the process of managing contracts.
Conclusion
In conclusion, machine learning is a rapidly evolving technology that is being used by the logistics industry to automate and improve various operations. It can be used to reduce errors, optimize delivery routes, and make accurate predictions. Though there are challenges associated with implementing machine learning systems, these can be overcome with the right planning and resources. As the technology continues to evolve, more and more applications are likely to arise, making machine learning a game-changer for logistics operations.
What is machine learning in Logistics?
Machine learning in Logistics is a form of technology utilized in the supply chain sector to improve the efficiency of operations, reduce costs, and maximize competitive advantage. This technology applies algorithms to large amounts of data generated in the supply chain to automate and optimize logistics processes, including inventory management, route optimization, and demand forecasting.
What types of data can be used for machine learning in Logistics?
Machine learning in Logistics is typically applied to data sets such as historical orders and shipment records, geospatial data, training data for predictive models, and other types of operational data.
How can machine learning in Logistics benefit my business?
Machine learning in Logistics can help businesses to increase their operational efficiency, reduce costs, and cut down delivery times by optimizing their supply chain processes. It can also help increase cost-saving opportunities by providing insights from data analysis that can inform better decisions about inventory management, pricing strategies, and route selection.